Pitfalls in PennCNV HMM
Many studies using PennCNV to predict CNVs observed a large number of false positives. This can happen by many factors, here we describe some of the most relevant ones that are responsible for the majority of false positive observed in iPSYCH project. Variation in hybridization intensity can mislead methods suggesting a CNV, genomic regions with high GC content are more likely to have lower hybridization as energy necessary for DNA denaturation is higher. Therefore, is common to observed those regions with lower Log R ratio than the rest of the chromosome. A lower value for LRR, when compared to the rest of the chromosome, will suggest a deletion. However, for a deletion to be true its BAF should be homozygous (BAF values 0 or 1). Some noise in BAF can create false heterozygous values (> 0 and < 1), but in high quality samples the amount of noise is below 5%. Therefore, any method to predict CNVs should be able to avoid false positive when LRR deviates from expecting using the heterozygosity level to control for false positives. Unfortunately, PennCNV model does not use well BAF information, allowding up to 25% heterozygosity in a deletion. This is often observed at telomeric and centromeric regions. To compensate for the poor model, it is suggested to remove those regions from the analysis, see at PennCNV user guide.
Example 1: When PennCNV model fail for deletion using real data.
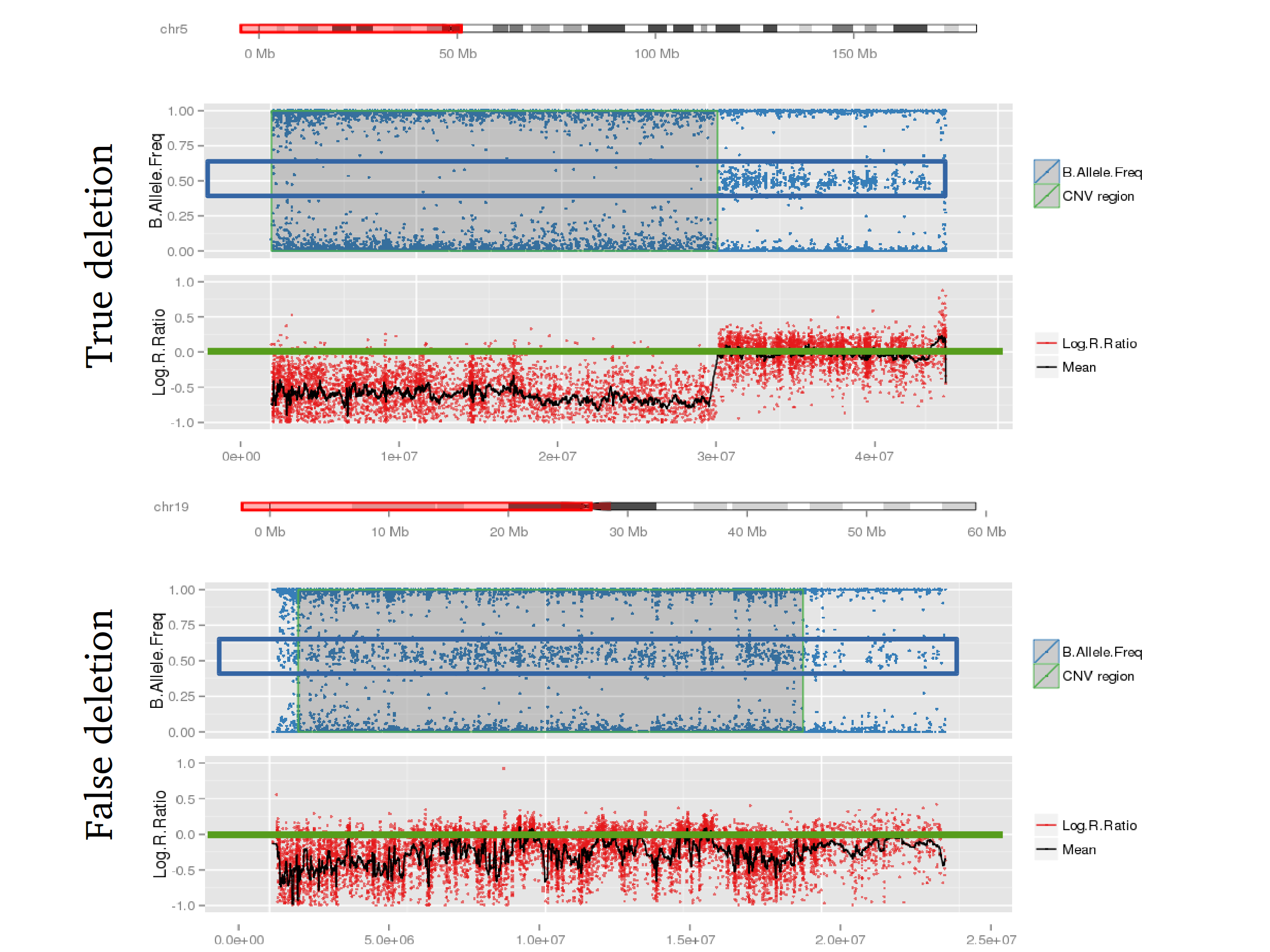
Example 2: When PennCNV model fail for duplication using real data.
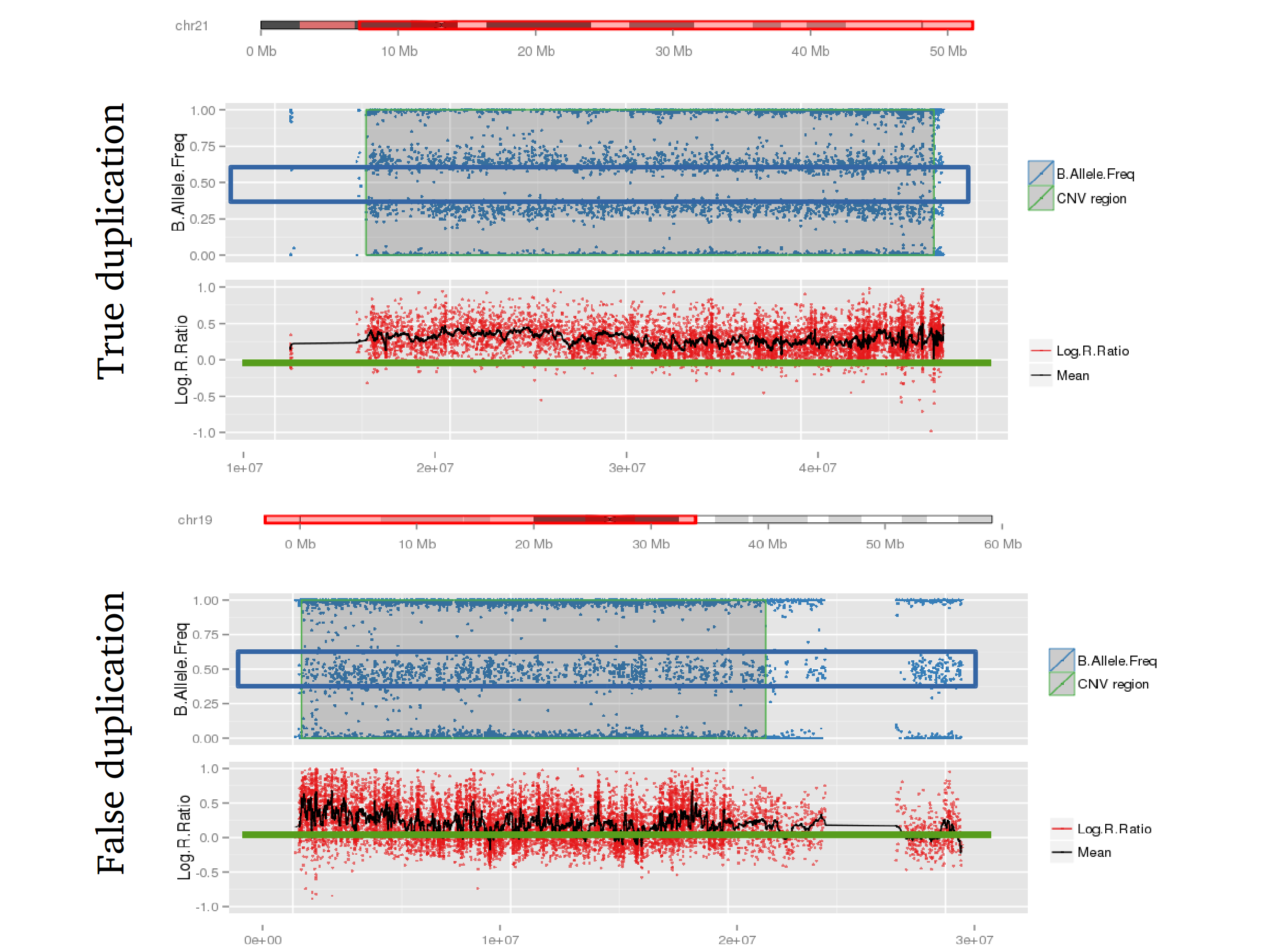